I am Simon, Han YANG! Currently I am a Ph.D. Candidate majored in Computer and Information Engineering(CIE) at Medical Micro Robotics Lab(MMRL), The Chinese University of Hong Kong, Shenzhen(CUHKsz) supervised by Prof. Zhuoran Zhang and Prof. Jiangfan Yu.
Before that, I received my B.S. degree from Hong Kong Baptist University and worked as a research assistant at Key Laboratory for Artificial Intelligence and Multi-Modal Data Processing of Department of Education of Guangdong Province in Beijing Normal-Hong Kong Baptist University(BNBU) working with Prof. Amy Hui Zhang until 2023.
My research interests include robotics micromanipulation system, cell surgery, 3D Visual Perception.
You can email me at hayang12 [at] link [dot] cuhk [dot] edu [dot] cn.
News
Publications
A method for estimating the depth information of motile cells and a robotic micromanipulation device [CN202411923420.7]
The present invention relates to the fields of robot technology and image processing technology, specifically referring to a method for estimating the depth information of motile cells and a robot micromanipulation device.
Weakly-Supervised Depth Completion during Robotic Micromanipulation from a Monocular Microscopic Image
This paper aims to address the challenge of acquiring dense depth information during robotic cell micromanipulation. A weakly-supervised depth completion network is proposed to take cell images and sparse depth data obtained by contact detection as input to generate a dense depth map.
Depth Estimation for Motile Cell under Monocular Microscopy Based on Fine-grained Classification
This paper models depth estimation as a multi-class classification task and introduces a fine-grained feature extraction block to discern subtle distinctions between focal planes.
HQNet: An Efficient Convolutional Neural Network for Cervical Cancer Classification
In this paper, an efficient deformable convolutional neural network system(HQNet) was constructed to identify Cervical Cancer cells with different degrees of development. A framework for bringing children's drawings of human figures to life.
WTBNeRF: Wind Turbine Blade 3D Reconstruction by Neural Radiance Fields
The method we use, called WTBNeRF is a network dedicated to wind turbine scene rendering and 3D reconstruction of wind turbines.
Experience
Research Intern
Robotic Micromanipulation System, IVF, Cell Surgery, 3D Visual Perception
3D Reconstruction and Computer Vision.
Research Intern
Research on Medical Image Processing for Cervical Cancer image classification.
Python Engineer Intern
Python Data Analysis, NLP, Cloud Database.
Posts
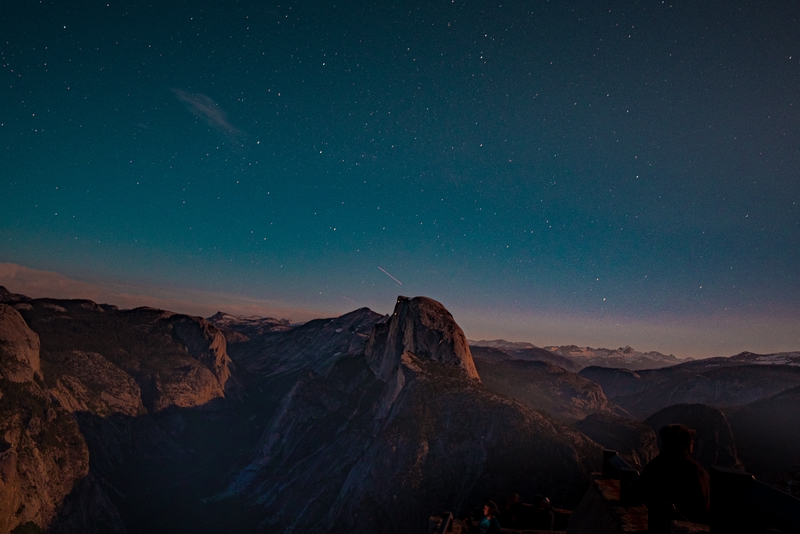
Helpful Resources
A growing resource list that might be useful to researchers and graduate students. I plan to update this frequently.